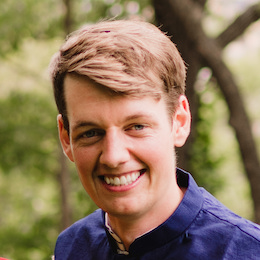
Jacob Calvert
I’m interested in collective behavior, which I study using probability theory and data science. Lately, I’ve been working on a principle of nonequilibrium self-organization and investigating how the behavior of a collective depends on the number of its constituents.
I’m a postdoctoral fellow at the Institute for Data Engineering and Science at Georgia Tech. I’ll spend Spring 2025 as the Berlekamp postdoctoral fellow at the Simons Laufer Mathematical Sciences Institute in Berkeley, CA.
For details on my academic background and experience as a professional data scientist, see About. For more on my research, check out Papers or Posts.
A new preprint with Shunhao Oh and Dana Randall explains how to program simple computational “particles” of different types to self-organize into corresponding spatial regions that have a given hierarchical structure. The size, shape, and hierarchy of the regions are determined by the particles’ densities and affinities for particles of different types. Proving that the algorithm works requires new techniques for analyzing the Gibbs distributions of fixed-magnetization models from equilibrium statistical mechanics.
Dana Randall and I generalize and prove the main claims of an emerging physical theory of nonequilibrium self-organization, called rattling theory, by interpreting its main claims in terms of Markov processes. The result is a principle that relates a local aspect of the dynamics to the global steady state. We apply it to random walks on random graphs, spin-glass dynamics, and models of ant colonies.
Clinical risk scores can cheat the AUC when the time that an adverse event occurs in a positive-class stay tends to exceed the duration of a negative-class stay. Risk scores should be compared to uniformly random scores that match their timing.